AI breakthrough: Predicting child survival after resuscitation
Dutch scientists developed a machine-learning model that can predict a child's survival chances within 24 hours after resuscitation.
Published on November 21, 2024
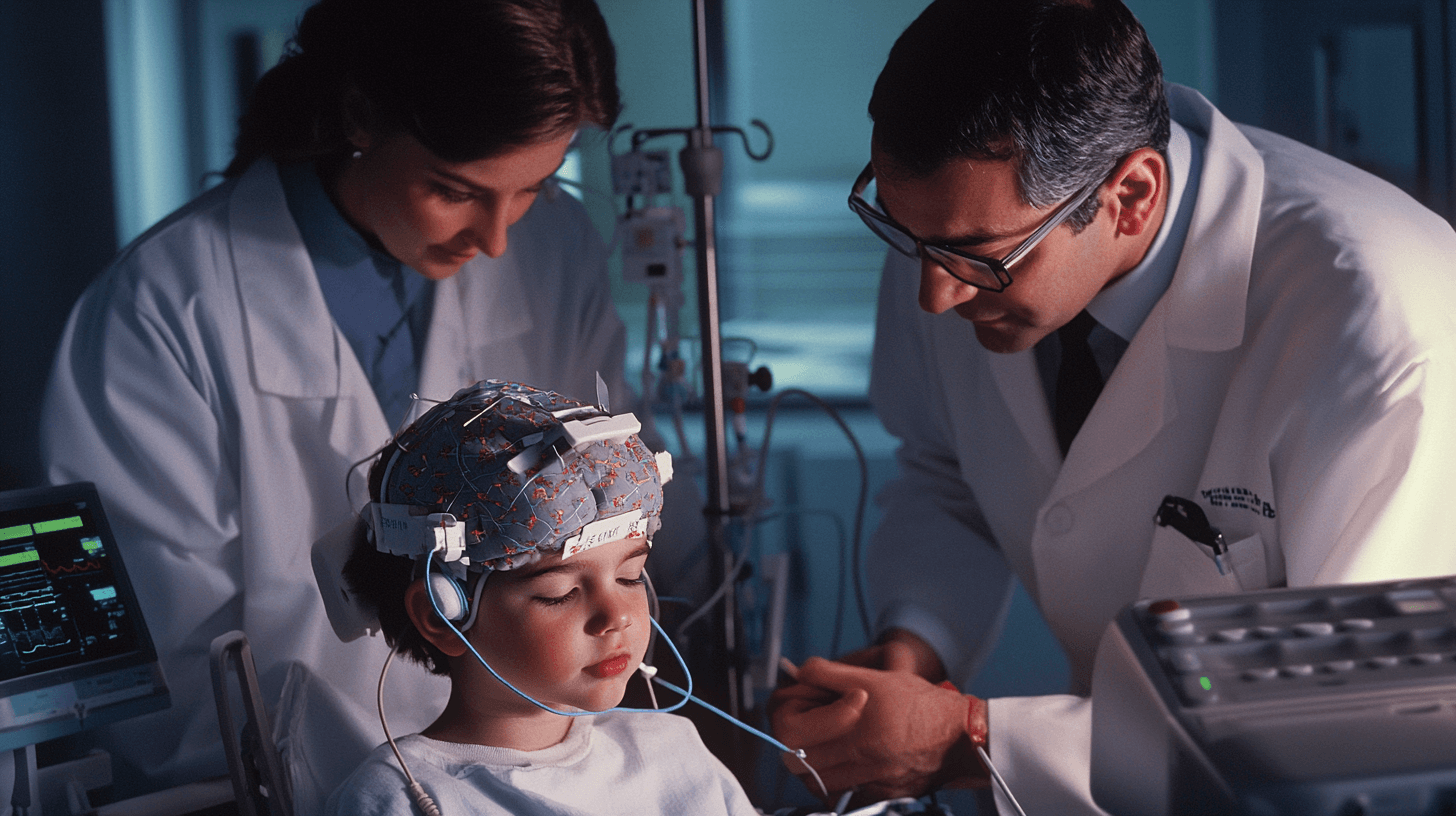
AI-generated image
Researchers from Erasmus Medical Center (Erasmus MC) and Delft University of Technology (TU Delft) have developed a machine-learning model that can predict a child's survival chances within 24 hours after resuscitation. This innovative tool, based on electroencephalogram (EEG) brain activity patterns, aims to provide crucial guidance to parents during an emotionally challenging time. While the model shows 100% accuracy in predicting death, it's still being refined for survival predictions. Currently undergoing testing in other hospitals, this collaborative effort between medical and technical experts could revolutionize post-resuscitation care and decision-making, offering hope and support to families facing uncertain outcomes.
Understanding cardiopulmonary resuscitation (CPR)'s critical role in child resuscitation is essential for appreciating the model's impact. CPR is a life-saving procedure initiated when a child's heartbeat or breathing ceases, commonly due to incidents like drowning or choking. Permanent brain damage can occur within minutes without blood flow, emphasizing the importance of rapid intervention.
In the critical hours following a child's resuscitation, parents face an agonizing wait for clarity about their child's future. The interval is fraught with uncertainty, a burden nearly as heavy as receiving bad news itself. Understanding whether a child will recover and to what extent is vital for making informed decisions about care and treatment.
The Erasmus University model addresses this need head-on, offering predictions based on EEG data that can be gathered swiftly after resuscitation. The project brought together experts from Erasmus MC and TU Delft, with key contributions from neurologist Robert van den Berg and statistician Geurt Jongbloed, alongside master's student Femke Lückerath.
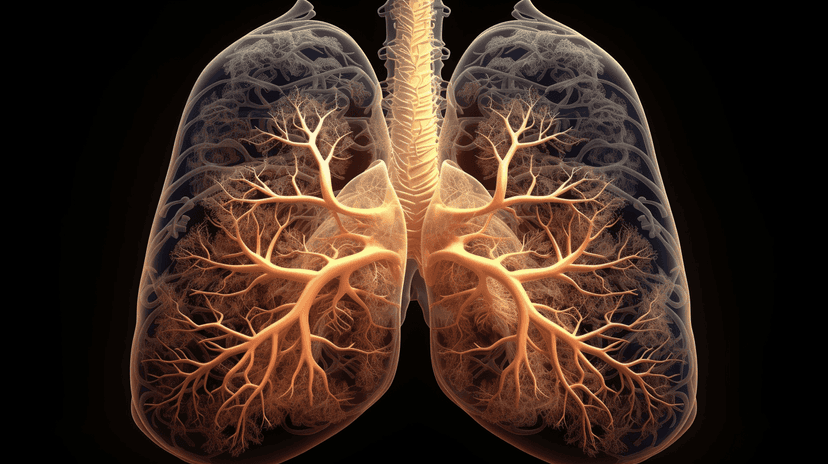
Saliva shows severity of recurrent respiratory infections in children
RadboudUMC: Measurement without blood sampling helps diagnosis and treatment
The science behind the model
The model employs machine learning to analyze EEG patterns, specifically looking for 'stills' in brain activity, which have been identified as significant indicators of poor outcomes. This method provides a more reliable forecast compared to adult cases, where patterns are less pronounced. The model's development involved training on data from resuscitated children, assessing health outcomes over a year, and refining algorithms to enhance predictive accuracy.
Despite its promise, the model currently excels in predicting death with 100% accuracy but struggles with survival predictions. Researchers are focused on understanding the model's decision-making process, aiming to improve its reliability. This involves ongoing hospital testing to ensure its robustness across different settings. The goal is to extend the model's capabilities to predict long-term development and tailor optimal treatment strategies for surviving children.
Empowering parents and caregivers
Beyond its scientific merit, the model offers practical support to families during distressing times. By providing predictions, it empowers parents with information that can guide emotional and medical decisions. As Van Den Berg points out, the model's insights can offer comfort, helping families navigate the toughest periods of their lives. This aligns with broader efforts to educate parents and caregivers about CPR, emphasizing preparedness for emergencies.